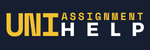
DATA6000 Capstone: Industry Case Studies Report Sample
Assignment Details
YourTask
Generate a unique business question that can be explored using available data sources and analytics methodologies mastered in the business analytics degrees.
Instructions
The first part of any good project is a literature review. In approximately 1000-1500 words (excluding references) address the following sections that will assist you to outline a business industry problem that can be addressed through data analytics.
1. Industry Background:
Chose an Industry, e.g., healthcare, retail, education, finance, recreation, government, etc. and discuss three key business problems currently facing this industry.
2. Existing Analysis and Methodologies:
Investigate and evaluate existing analysis on the three business problems you have chosen and reflect on the data and analytics methodologies that have been employed this analysis.
3. Data Sources:
Evaluate the types of data sources available to analysts in this industry. Explore the available data sources you can access to address the three business problems you have identified and evaluate what type of descriptive and predictive analytics techniques could be used.
4. Selecting Business Problem:
Generate a unique business question for this industry based on one of the business problems. You will address this business problem in Assessment 3 (Project Report).
For the business problem you select briefly summarise the:
1. The data source you will use.
2. Methodologies you will explore (brief).
3. The originality of your contribution (i.e. Why your is analysis unique given existing investigation and analysis in this space?).
5. Provide at least ten relevant, credible references to support your ideas and explanations.
Solution
Executive Summary
The report illustrates the investigation of a specific business problem which highlights the investigation of multiple business-related issues. The issues provide the organizational fault which is faced by different organizations. In this report, the focused sector is the hospitality management sector. This sector provides hospitality to all the users. The functionality defines the involvement of the logical factors which is used to investigate the collected dataset. The examination process defines the implementation of a data visualization process which is used to visualize the data in a suitable way. The approach defines the implementation of the logical factor which is used to check the functionality of multiple factors of the system. The process defines the involvement of the time and date-wise analysis. Here the hospitality system is analyzed with the help of hotel booking details data. This data is used to investigate the demand of the hotel among multiple customers.
The investigation process also defines the use of logical factors which are used to process the data in a suitable manner. The processing of the data illustrates the data evaluation process which is used to evaluate the data by using a suitable logical process. The process defines the evaluation of the data in a suitable manner. The logical process defines the evaluation of the data in a proper manner for university assignment help.
Industry Problem
The hospitality industry's cost of living challenges demands a multifaceted analytical approach. Analyzing consumer spending patterns through data on discretionary expenses and inflationary pressures is key. Understanding how rising food and energy prices impact customer behavior is vital. Labor costs, supply chain disruptions, and operational expenses require detailed data scrutiny. To address this, comprehensive analytics methods integrating customer behavior analysis, cost trend monitoring, and operational efficiency metrics are essential (Kansakar et al., 2019). Robust analytics can aid in implementing targeted cost control measures, optimizing operations, and mitigating risks caused by escalating costs, fostering resilience in a volatile market.
The evolving consumer landscape in the hotel sector demands a nuanced approach to analytics and data utilization. To address supply chain challenges, analyzing historical data on cost fluctuations, supplier reliability metrics, and seasonal demand patterns is crucial. Labor cost optimization requires predictive analytics for staffing needs, employee retention strategies, and adaptive marketing insights. Understanding guest preferences mandates sentiment analysis of reviews and customer data. Innovating involves leveraging market segmentation analytics to target untapped demographics and tailoring services (Amalina et al., 2019). Implementing robust data security measures and technology investments is imperative. Adapting analytics to optimize operations, manage costs, and foresee trends ensures resilience and sustained growth in this dynamic industry.
Data Sources (and methodology potential)
.png)
(Source: Orange)
The structure of the dataset defines the implementation of the structural-functional data. This defines multiple data variables such as booking data, hotel details, customer data, and so on. All those data are used in the data investigation process which defines the calculative approach in the data evaluation process. The functionality defines the evaluation of the data with the help of the numeric data value investigation process as well as the object data value investigation process.
Figure 2: Data Prediction Model 1
(Source: Orange)
The data prediction model 1 illustrates the implementation of the data in the main structural model. This model is used to display the tree structural value of the model which has a specific prediction data value. The predictive value defines the evaluation of the tree structure model. It also illustrates the use of the scatter plot as well as matrix which is the main analytical part of the investigation.
Figure 3: Predictive value
(Source: Orange)
The predictive value evaluation process demonstrates the evaluation of the ‘Tree’ model prediction value. This illustrates the use of the AUC, CA, F1, Prec, Recall, and MCC. As per the investigated outcome, the predictive F1 value for this model is 1.
Figure 4: Scatter plot between lead time and adr
(Source: Orange)
The scatter plot between two major data variables is displayed in this section. It highlighted the evaluation of the relation between lead time, and adr. The evaluation assists in understanding the relation between those two factors. It also defines the implementation of two types of adr values such as 0, and 1.
Figure 5: Confusion Matrix
(Source: Orange)
The matrix illustrates the use of a confusion process which highlights the relation between actual and predicted data values. This defines the relation between 0, and 1 data types which is the main part of the investigation (Haoxiang, and Smys, 2021).
Figure 6: Data Prediction Model 2
(Source: Orange)
The second predictive model defines the use of linear regression which is another important model. This model defines the use of the predictive technique and approach which assist in finding out the linear regression investigation data. The process also defines the use of the scatter plot, as well as the descriptive analytical plot.
Figure 7: Linear regression model details
(Source: Orange)
Linear regression model details are illustrated in this section. It illustrates the functionality of the implementation of multiple logical parameters of a system. This also defines the impact of the linear regression in the data analysis process.
Figure 8: Scatter plot for model 2
(Source: Orange)
The scatter plot for model 2 is illustrated in this portion. It defines the evaluation of lead time vs adr with respect to the calculation factors. This also demonstrates the implementation of different factors as well as parameters.
Figure 9: Distribution of lead time data
(Source: Orange)
The distribution of the lead time data is illustrated in this portion. It defines the examination of the frequency distribution process. The evaluation defines the execution of the data in a proper way (Church et al., 2019).
Figure 10: Distribution of hotel data
(Source: Orange)
The hotel data distribution is illustrated in this portion. This defines two types of hotel distribution data such as ‘City Hotel’, and ‘Resort Hotel’.
Figure 11: Distribution of meal data
(Source: Orange)
The distribution of the meal data is also illustrated in this portion. This defines the involvement of multiple meal values such as BB, FB, HB, and SC (Wang et al., 2022).
Figure 12: Distribution of Reservation Status data
(Source: Orange)
The distribution of the reservation status data is illustrated in this portion. This defines the evaluation of the canceled, no-show, and check-out data.
Selecting Business Problem
Cost of living
The hospitality industry is facing a cost-of-living challenges due to several barriers. The hospitality sector is heavily reliant on discretionary spending; therefore, it is often one of the major to feel the impact of people tightening their budgets. It also identified that the cost-of-living crisis is causing inflationary pressures that are changing customer spending habits. Customers are now more careful with their spending. Some of the vital cost of living problems which mainly faced by the hospitality industry include Food prices, energy prices, Labor costs, ingredients, Supplies, Utilities, Operational costs (Kaushal, and Srivastava, 2021).
Apart from these, the hospitality industry is facing a living cost problem due to several factors such as Rising food prices, “increased energy prices”, “inflationary pressures”, “Customers spending less”. The entire hospitality industry was one of the major hits due to the global pandemic. Rising costs are putting jobs and businesses at different risks. Efficient cost control measures “streamline operational processes within the hospitality industry”.
Changing Consumer Preferences
The changing tastes of consumers are bringing about a revolutionary scenario for the hotel sector. Traditional hotel models have been affected by alternative lodgings such as home-sharing platforms and holiday rentals. This change necessitates industry adaptation. Operational efficiency is impacted by supply chain management challenges with cost control, supplier dependability, and seasonal variations. One major issue that hurts profitability and competitiveness are labour expenses. Complexity is increased by hiring, keeping employees, and adapting to changing marketing trends.
The industry may innovate to meet these issues. It is essential to adopt novel approaches and business methods, customize services for devoted customers, and draw in unexplored market niches. Understanding and catering to changing guest expectations, optimizing operations, managing rising consumable costs, and ensuring data security are crucial adaptations (Handfield et al., 2019). By embracing flexibility, redefining service standards, implementing robust supply chain solutions, and investing in technology, the hospitality sector can navigate these challenges. This approach fosters resilience, facilitates market relevance, and ensures sustained growth in an ever-evolving consumer-centric landscape.
Housekeeping Issues
In the hospitality industry, housekeeping issues can pose multifaceted challenges. Hazards like exposure to cleaning chemicals and infectious agents from linens or bodily fluids demand stringent safety measures. Slips, trips, and fatigue stemming from long hours add to these concerns, affecting staff health and productivity. Operational hurdles arise from high turnover rates, insufficient data, and adapting to new technology. Meeting escalating guest expectations while maintaining stringent cleanliness standards presents a constant challenge. Neglecting any aspect of cleanliness, disregarding guest complaints, or allowing clutter in shared spaces can tarnish a hotel's reputation and profit margins (Kansakar et al., 2019). Issues such as low-quality furnishings, broken amenities, dampness, and overlooked cleanliness details hair, rubbish, fingerprints, and dust directly impact guest satisfaction and consequently, a hotel's bottom line. Addressing these multifarious challenges requires a proactive approach, effective training, and a commitment to excellence in hospitality services.
Reference List
Kaushal, V. and Srivastava, S., 2021. Hospitality and tourism industry amid COVID-19 pandemic: Perspectives on challenges and learnings from India. International journal of hospitality management, 92, p.102707. https://www.sciencedirect.com/science/article/pii/S0278431920302590
Han, H., 2021. Consumer behavior and environmental sustainability in tourism and hospitality: A review of theories, concepts, and latest research. Journal of Sustainable Tourism, 29(7), pp.1021-1042. https://doi.org/10.1080/09669582.2021.1903019
Kansakar, P., Munir, A. and Shabani, N., 2019. Technology in the hospitality industry: Prospects and challenges. IEEE Consumer Electronics Magazine, 8(3), pp.60-65. DOI: 10.1109/MCE.2019.2892245
Amalina, F., Hashem, I.A.T., Azizul, Z.H., Fong, A.T., Firdaus, A., Imran, M. and Anuar, N.B., 2019. Blending big data analytics: Review on challenges and a recent study. Ieee Access, 8, pp.3629-3645.
Haoxiang, W. and Smys, S., 2021. Big data analysis and perturbation using data mining algorithm. Journal of Soft Computing Paradigm (JSCP), 3(01), pp.19-28. 10.36548/jscp.2021.1.003
Church, S.P., Dunn, M. and Prokopy, L.S., 2019. Benefits to qualitative data quality with multiple coders: Two case studies in multi-coder data analysis. Journal of Rural Social Sciences, 34(1), p.2. https://egrove.olemiss.edu/jrss/vol34/iss1/2/
Handfield, R., Jeong, S. and Choi, T., 2019. Emerging procurement technology: data analytics and cognitive analytics. International journal of physical distribution & logistics management, 49(10), pp.972-1002. https://doi.org/10.1108/IJPDLM-11-2017-0348
Wang, J., Xu, C., Zhang, J. and Zhong, R., 2022. Big data analytics for intelligent manufacturing systems: A review. Journal of Manufacturing Systems, 62, pp.738-752. https://doi.org/10.1016/j.jmsy.2021.03.005