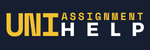
DATA4400 Data-driven Decision Making and Forecasting Report 3 Sample
Your Task
Develop a real-world forecasting project plan/proposal based on the learnings from the subject.
Assessment Description
This assessment seeks to simulate a real-world task that you may have to undertake in the future. Therefore, the assignment is non-prescriptive and requires you to pose a relevant, small, creative and significant problem to solve that could result in benefits to the organisation of choice.
In this assessment, you need to consider an organisation in an industry of your choice and articulate the steps this organisation needs to take to enable forecasting for data-driven decision making. You are required to analyse a sample data set to demonstrate expected forecasting outcomes.
You need to be familiar with the organisation and industry (e.g., where you have worked or areworking, a future start-up company), NOT an organisation such as Amazon/Boeing/Qantas etc.
The report should address:
o Why forecasting would help this organisation given their current operations.
o The forecasting technique(s) you would recommend.
o Justification of your recommended forecasting technique(s) by analysis of a relevant sample dataset.
o The benefits of this forecasting project for the organisation (The benefit could be financial, such as Return on Investment (ROI) or societal benefits).
Assessment Instructions
• By Week 9 identify a company and industry you are familiar with that would benefit from a forecasting application. Note:
o The application needs to be based on forecasting (not some other aspect of analytics).
o Focus on a single, well defined (small) application.
o Sample datasets maybe sourced from:
? an organisation if you work there,
? public repositories such as kaggle.com and https://archive.ics.uci.edu/ml/datasets.php,
? Open government data such as abs.gov.au.
• By Week 12 draft some preliminary points pertaining to the report in class. You are encouraged to consider the current mode of operation, possible inefficiencies, available data and how this data may be used to provide efficiencies based on the concepts and techniques covered in the subject. Think of yourself as a consultant or a founder.
• Your lecturer will advise on the appropriateness of your choice and proposed methodology regarding the requirements for the assessment.
• Include a list of references that is directly related to the content. Each reference needs to be linked to at least one specific point in the content of your assessment. It is expected that you will have at least 10 relevant references.
• Discuss or show how ChatGPT can be leveraged to enhance your solution.
Solution
Introduction:
In today's rapidly evolving business landscape, the ability to make data-driven decisions is paramount. As organisations seek to harness the power of data analytics, understanding the key factors influencing successful implementation becomes crucial. This study delves into the critical components of effective data-driven decision-making in contemporary business environments. By examining the various approach of forecasting, this research aims to provide valuable insights for businesses navigating its business challenge. Through focusing on the issue of General Electric (GE), present study has been done, which not only has showcased the use of forecasting, additionally has demonstrated use of artificial intelligence regarding streamlining the research work.
Business Challenge:
General Electric (GE) grapples with several critical challenges in its financial performance. One of the foremost concerns is the stark decline in its revenue, plummeting from $149,567 million in 2010 to a mere $76,555 million in 2022 (macrotrend.net 2023). Additionally, GE grapples with a formidable challenge in the form of its increasing debt burden. The company's long-term debt swelled to a staggering $97,580 million in 2019, a figure nearly equivalent to its market capitalisation of $94,100 billion (macrotrend.net 2023). This exorbitant debt load has imposed severe limitations on GE's capacity to invest in promising growth opportunities and has led to substantial interest obligations, further straining its financial resources. These factors are potential for influencing the share prices and in return share prices are influencing the market capitalisation and value of GE. GE faces the critical issue of a precipitous decline in its share price, which has plummeted from a robust $297.62 in July 2000 to a mere $111.47 in October 2023 (finance.yahoo.com 2023). This protracted decline in share price, coupled with the erosion of revenue, compounds the company's financial woes, necessitating strategic measures to address its persistent challenges and restore its financial health.
Benefit of forecasting for the organisation with their current operations:
Forecasting plays a significant part in helping associations to pursue informed and key choices, and it can significantly help an organisation like GE underpinning its ongoing tasks. Forecasting can furnish GE with significant experiences into its unpredictable financial scene. GE's presence in different areas, including flight, medical care, and sustainable power, one of which is vulnerable to unmistakable market elements and outside factors. By utilising forecasting models, GE can acquire a far-reaching comprehension of the expected variances, market patterns, and monetary circumstances intended for every one of its business fragments (Chambers et al. 1971). This granularity empowers the organisation to dispense assets, distinguish learning experiences, and relieve gambles by going with information driven choices custom fitted to the novel difficulties of every area.
Moreover, the capacity to forecast can fundamentally improve GE's financial planning and planning processes. The ongoing lower direction in share cost and income requires a fastidious assessment of the organisation's financial wellbeing and manageability. Precise forecasting can give GE's administration a clearer image of its future income streams, cost designs, and productivity (Chambers et al. 1971).
In addition to financial planning, forecasting can bolster GE's supply chain management. The company relies on intricate supply chains to source materials and deliver products and services across the globe. Accurate demand forecasts can enable GE to optimise its supply chain operations, reducing excess inventory, minimising lead times, and enhancing overall efficiency (Chambers et al. 1971). Besides, forecasting can aid GE in resource allocation and investment decisions. With declining share prices and revenue, it is crucial for the company to identify areas where investments can yield the highest returns (Talagala et al. 2023). By employing forecasting models, GE can prioritise projects and initiatives that align with market trends and future demand, thereby optimising its capital allocation and ensuring a more efficient use of resources.
Forecasting technique recommendations:
Given that the analysis is based on the close price data between November 2000 to October 2023, which is aggregated monthly, time series forecasting data technique is recommended for case organisation (finance.yahoo.com 2023). Time series analysis focuses on analysing and forecasting data points collected or recorded over a period, making it particularly relevant for GE’s situation (Shah et al. 2019). There is various approach of performing forecasting which can be time series-based forecasting, regression analysis and trend prediction. For time series forecasting like trend analysis, exponential smoothing, Autoregressive Integrated Moving Average (ARIMA) model, Vector Autoregression (VAR) model and Seasonal Autoregressive Integrated Moving Average (SARIMA) model are widely used approach (Strader et al. 2020).
ARIMA model is one of the best choices for forecasting that case organisation can utilise. This model is ideal for time series data, making them perfect for analysing GE's monthly closing share prices. ARIMA models are ideal for forecasting GE's share prices as they can handle seasonality, identify trends, and require data stationarity. This is important for GE, which operates in multiple industries with distinct market cycles and has recently experienced share price volatility. ARIMA models can help GE make informed decisions about resource allocation, growth opportunities, and risk mitigation (Strader et al. 2020).
Trend analysis and SARIMA and VAR models are valuable tools to complement ARIMA modelling for forecasting GE's share prices. Trend analysis provides a qualitative understanding of the long-term dynamics affecting share prices, such as industry trends, regulatory changes, and economic conditions. SARIMA models explicitly address seasonality, which can be a significant factor in share price movements (Gromann 2019). VAR models enable multivariate analysis, allowing GE to explore the interdependencies between share prices and other time series variables, such as revenue. This holistic view of variable interactions can help GE gain a deeper understanding of the broader financial dynamics at play and identify causal relationships between share prices and revenue (Obthong et al. 2020).
Another recommended method of forecasting is exponential smoothing. Exponential smoothing can be incorporated into GE’s forecasting strategy instead of ARIMA, trend analysis, SARMA, and VAR due to its unique attributes. Exponential smoothing models excel in capturing trends and seasonality in time series data, which is essential for understanding share price fluctuations. Their adaptability and the use of smoothing coefficients allow GE to fine-tune the model to changing data patterns, ensuring accurate forecasts. Additionally, these models are particularly well-suited for short to medium-term forecasting, aligning with GE's need to predict near-future share prices (Madhavan 2019). By integrating exponential smoothing into their forecasting toolkit, GE gains a comprehensive approach that addresses both quantitative and qualitative aspects of financial forecasting, empowering them to make well-informed decisions in the face of falling share prices and revenue.
Justification of chosen forecasting technique:
The utilisation of the Exponential Smoothing forecasting model, guided by the quality metrics and smoothing coefficients, underscores its efficacy as an advantageous technique for forecasting GE monthly close share prices (Strader et al. 2020). This validation is grounded in a meticulous analysis of a pertinent sample dataset, revealing the model's adeptness in capturing essential nuances such as underlying trends, seasonality, and providing forecasts with commendable accuracy. As the data has no obvious trend and seasonality, hence consideration of exponential smoothing is considered here. VAR model is not valid here as only single variable has been considered for analysis. Moreover, ARIMA and SARIMA fails to apply here as value of alpha, beta and gamma is not known here.
Data source:
To forecast, data of case organisation close price has been considered. Monthly data for July 2020 to October 2023 data has been collected from yahoo finance (finance.yahoo.com 2023).
Methodology:
To do model forecasting, tableau has been used. Forecasting is done without any seasonality and trend as data does not showcase specific seasonality or trend as can be seen from figure 1. Using RMSE, MASE and AIC, model validation has been checked.
Figure 1: Forecasting finding
As per the figure 1, no clear trend in close price of GE can be observed. Neither seasonality is observable from the above figure. Hence, forecasting of next 3 months has been made using the exponential smoothing here.
Figure 2: Forecasting
As per the figure 2, forecasting shows a slight drop in share price in November, with rise in December and again falling trend in February can be observed.
Figure 3: Forecasting statistics
Based on the figure 3, Root Mean Square Error (RMSE) found to be 10.4. RMSE serves as a pivotal yardstick of forecasting precision. The Exponential Smoothing model, with an RMSE of 10.4 offers forecasts that closely align with actual close prices. This means, on average, the model's forecasts deviate by approximately $10.4 from the factual close prices (Magiya 2019). Considering the inherent volatility of share prices, this level of accuracy is indeed noteworthy. Apart from this, MASE or Mean Absolute Scale Error is a relative measure of forecasting accuracy, showcases the superiority of the Exponential Smoothing model in comparison to a naive forecast (Magiya 2019). A MASE value below 1 implies the model's outperformance. In this scenario, a MASE of 0.34 signifies that the Exponential Smoothing model's forecasts are merely 0.34 times as volatile as those derived from a naive forecast. This emphasises the model's superior predictive capabilities (Magiya 2019).
As per the calculated value, Alpha found to be .5. Exponential Smoothing model employs an equal weighting approach to the most recent observed close price and the previously predicted level. This balanced approach adeptly captures the level or baseline of the time series, reflecting the model's ability to adapt to changing data patterns. The value of 0.000 for beta indicates that the model does not incorporate a trend component at present (Magiya 2019). While this might suffice for stationary data, it may necessitate reconsideration in the future if there is an evident emergence of long-term trends in GE's share prices. A gamma value of 0.247 underscores the model's consideration of the seasonal component, albeit with some smoothing. This is invaluable for capturing recurrent seasonal patterns inherent in financial data due to market cycles.
Benefit of exponential smoothing forecasting technique to the organisation:
Forecasting is very important for understanding the trend and seasonality to predict the potential future change in share price. As share price is directly linked with the firm’s revenue, hence potential increase in share price can increase the revenue as well.
Through the time series analysis, GE can reveal historical patterns and trends in share price movements. By examining past price behaviour, GE can gain insights into the factors that have influenced share prices in the past, helping to make predictions about future movements. When it comes to the exponential smoothing, the technique allows for the identification of seasonality and trends within the data. GE can analyse whether share prices exhibit regular patterns or trends related to specific months, quarters, or years (Cavalier 2020). This information is vital for forecasting future price movements accurately. Moreover, this model forms the baseline for the SARIMA model as it provides justified alpha, beta, and gamma values to further streamline the forecasting for shorter period. Hence exponential smoothing is the baseline approach that GE should consider for forecasting.
Use of ChatGPT to enhance solution:
Leveraging ChatGPT can enhance the solution by incorporating an AI-driven conversational interface. ChatGPT can facilitate real-time interaction with users, answering queries, providing insights, and explaining complex findings from the study. This interactive capability fosters a user-friendly experience, aiding in better understanding and application of the research outcomes. Additionally, ChatGPT can assist in data interpretation and offer on-demand assistance, making the study's insights more accessible and actionable for a broader audience, ultimately enhancing the value and usability of the research findings.
Conclusion:
To conclude, it can be stated that this study has illuminated the essential factors underpinning effective data-driven decision-making in contemporary business contexts. Valuable insights have been garnered by analysing the interplay of data quality, technology infrastructure, and organisational culture. The findings serve as a roadmap for organisations seeking to navigate the data-driven landscape successfully. In an era where data is invaluable, harnessing these insights is paramount to achieving a competitive edge and ensuring sustainable growth. As businesses continue to evolve, the ability to leverage data effectively remains a critical determinant of success, and this study paves the way for informed and strategic data-driven decisions.
Reference:
Cavalier, J-P 2020, Coronavirus (COVID-19) stats, Tableau Public, viewed https://public.tableau.com/profile/jean.paul.cavalier#!/vizhome/Coronavirus2019-nCoVstats/Coronavirus2019-nCoV
Chambers, JC, Mullick, SK & Smith, DD 1971. ‘How to choose the right forecasting technique’, Harvard Business Review, vol. 49, no. 4, Harvard Business Publishing, pp. 45–70, viewed http://search.ebscohost.com/login.aspx?direct=true&AuthType=shib&db=edb&AN=3866910&site=eds-live&custid=s1234057
Finance.yahoo.com 2023. General Electric Company (GE) Stock Historical Prices & Data (2023) Yahoo! Finance. Yahoo! https://finance.yahoo.com/quote/GE/history?period1=1602028800&period2=1696636800&interval=1mo&filter=history&frequency=1mo&includeAdjustedClose=true
Gromann, E 2019. ‘Forecasting in the age of big data’, Accounting today, vol. 33. https://www.accountingtoday.com/opinion/forecasting-in-the-age-of-big-data
Macrotrends.net 2023. General Electric Market Cap 2010-2023: GE (no date) Macrotrends. https://www.macrotrends.net/stocks/charts/GE/general-electric/market-cap
Madhavan, A., 2019. Correlation vs causation: Understand the difference for your product. https://amplitude.com/blog/2017/01/19/causation-correlation
Magiya, J 2019. Introduction to forecasting in data science, Medium publication. https://towardsdatascience.com/introduction-to-forecasting-in-data-science-676db9b55621
Obthong, M., Tantisantiwong, N., Jeamwatthanachai, W. and Wills, G., 2020. A survey on machine learning for stock price prediction: Algorithms and techniques. https://www.academia.edu/download/57743652/ICBAI_2018_Final_Paper.pdf
Shah, D., Isah, H. and Zulkernine, F., 2019. Stock market analysis: A review and taxonomy of prediction techniques. International Journal of Financial Studies, 7(2), p.26. https://www.mdpi.com/2227-7072/7/2/26/pdf
Strader, T.J., Rozycki, J.J., Root, T.H. and Huang, Y.H.J., 2020. Machine learning stock market prediction studies: review and research directions. Journal of International Technology and Information Management, 28(4), pp.63-83. https://scholarworks.lib.csusb.edu/cgi/viewcontent.cgi?article=1435&context=jitim
Talagala, T.S., Hyndman, R.J. and Athanasopoulos, G., 2023. Meta?learning how to forecast time series. Journal of Forecasting, 42(6), pp.1476-1501. https://robjhyndman.com/papers/fforms.pdf